Envise™
The World's First Photonic Computing Platform
The Problem
Reduce the carbon footprint and operating cost of your datacenter while powering the most advanced neural networks (and the next generation) with a fundamentally new, powerful and efficient computing platform: photonics.
The Solution
You can’t increase the power supply to the building, so you either build a new one or find better hardware. We enable your roadmap for bigger, more capable neural network models— increasing product margins and allowing you to deploy new features all while minimizing your environmental footprint.
It's Time for Something (R)evolutionary
Through the combination of electronics, photonics, and new algorithms, we’ve built a next-generation computing platform purpose-built for AI.
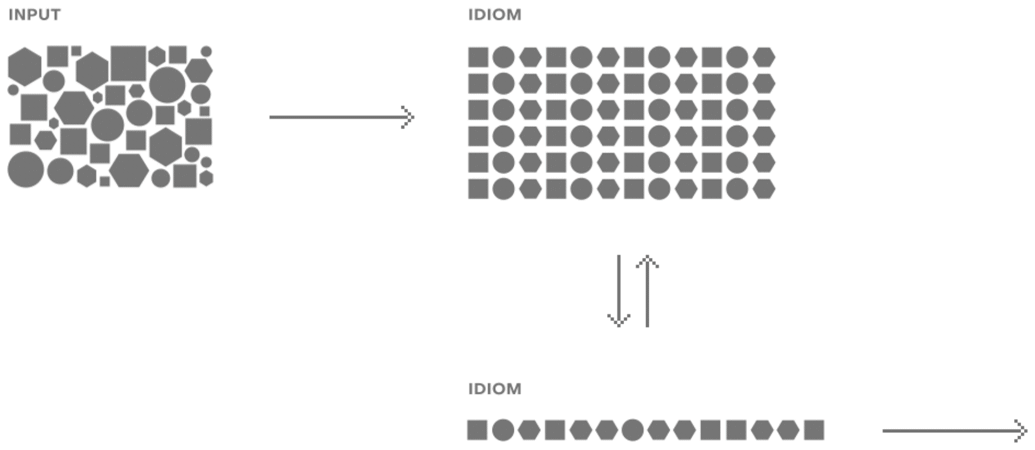
A Performance Scaling Paradigm
Photonics enables multiple operations within the same area.
Compute Density
Compute Speedup
Performance / Power
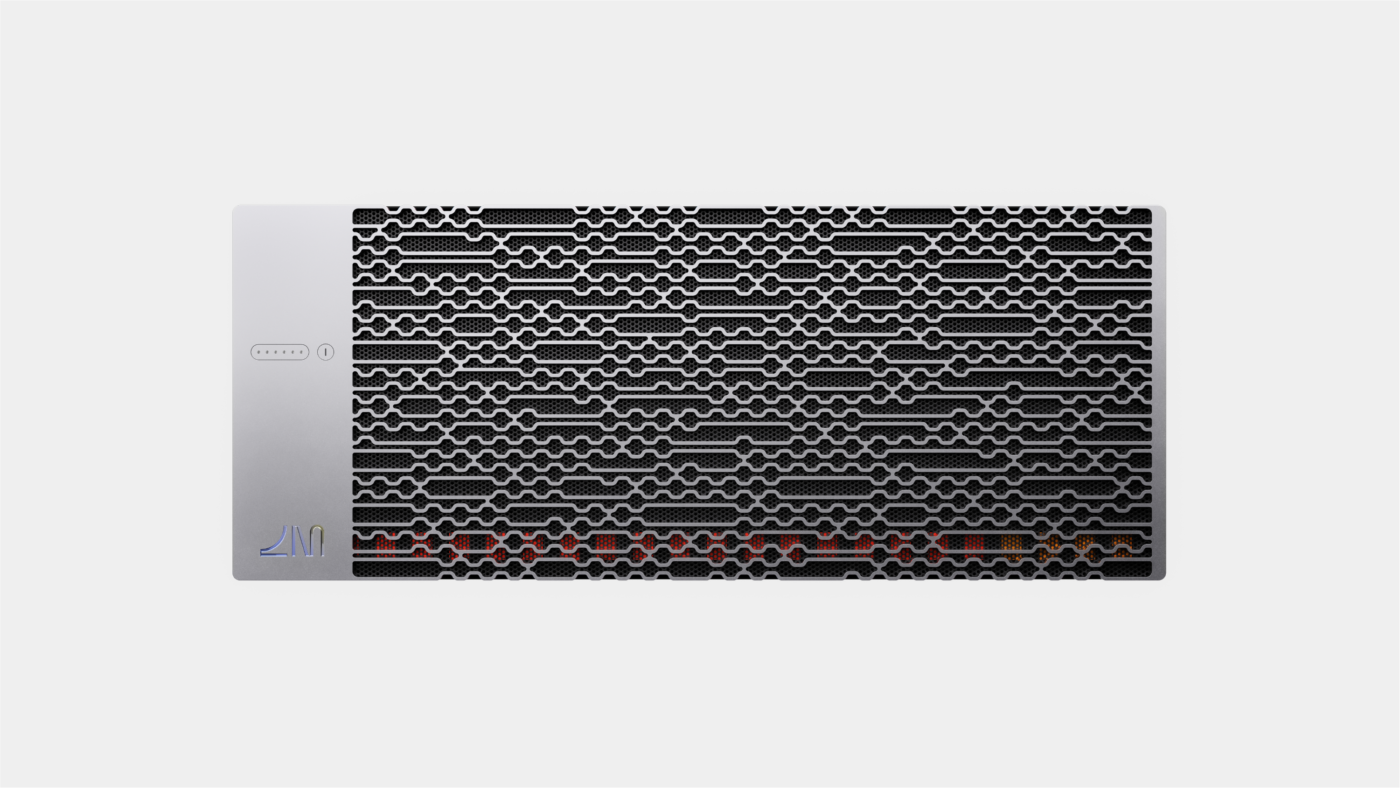
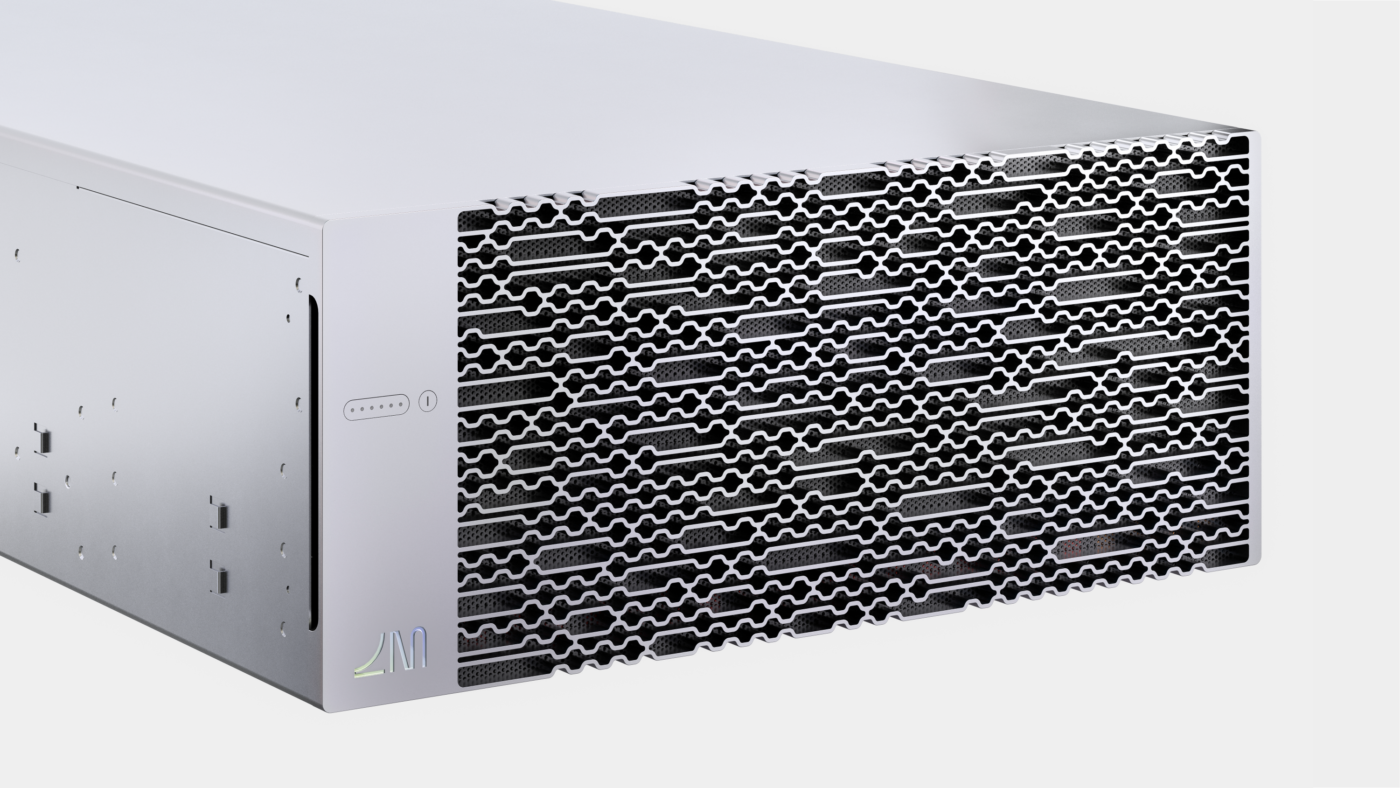
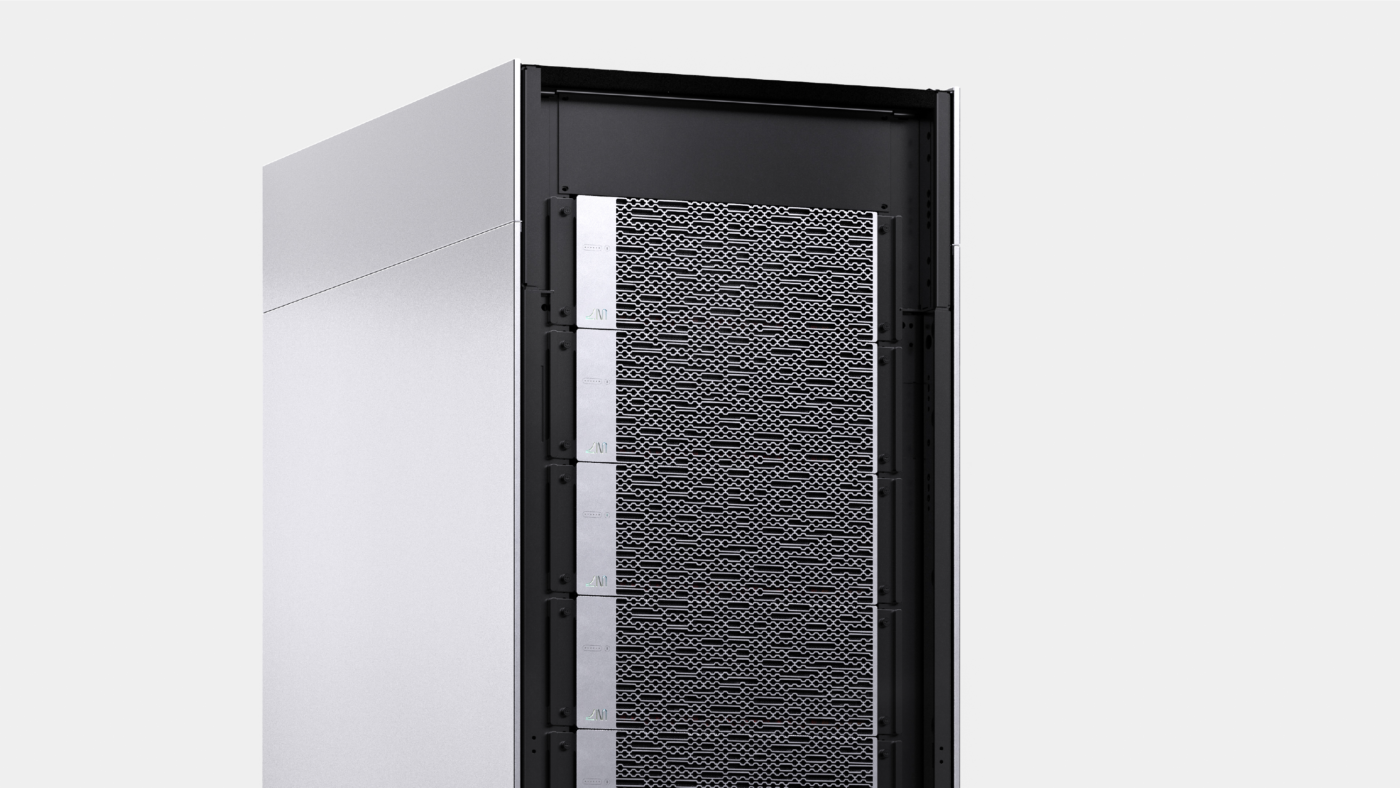
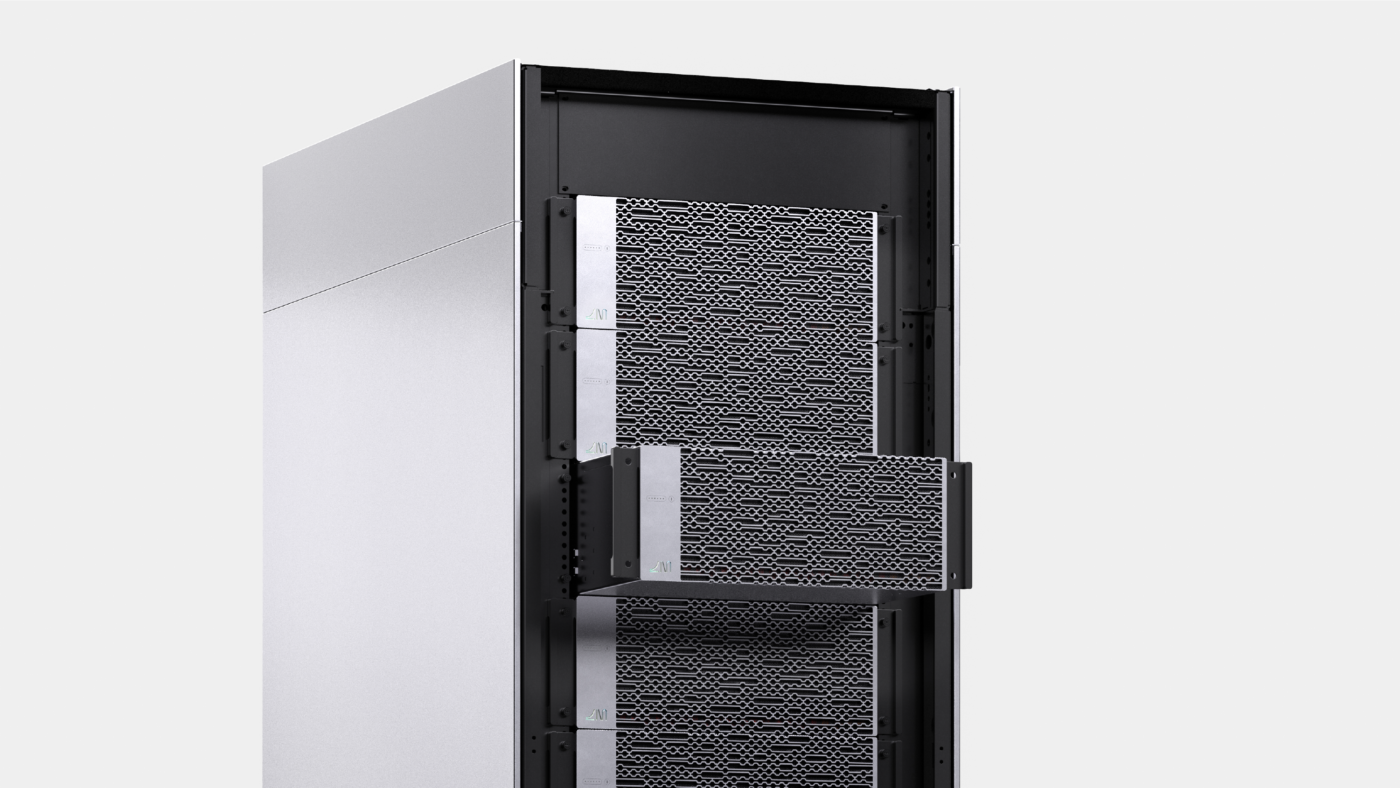
Specifications
Massive on-chip activation and weight storage enabling state-of-the-art neural network execution without leaving the processor.
Standards-based host and interconnect interface. Revolutionary compute, standard communications.
RISC cores per Envise™ processor. Generic off-load capabilities.
Ultra-high performance out-of-order super-scalar processing architecture.
Deployment-grade reliability, availability, and serviceability features. Next generation compute with the reliability of standard electronics.
400Gbps Lightmatter® interconnect fabric per Envise™ chip — enabling large model scale-out. Running the most advanced neural networks on the planet.
ENVISE™ Server
- 16xLightmatter® Envise™
- 2xAMD EPYC 7002 series host processors
- 3TB NVMe SSD
- 6.4Tbps LM-Fabric for scale-out
- 2x200G Ethernet Smart NIC
- Gigabit ethernet for IPMI management
- 3kW TDP
- 4U form factor
Auto
Autonomous Driving
Robotics
Vision and Control
E-Commerce & Advertising
Product Recommendation
Manufacturing
Predictive & Preventative Maintenance
Health
Pharmaceuticals
Pathology
Cancer Detection
Customer Service
Digital Assistants
Chatbots
Signal Processing
Analysis of Digital Signal
Algorithms
Natural Language Processing
Text-to-Speech
Language Translation